The AI Edge: Redefining Financial Services in the Age of Intelligence
22 min read
Artificial Intelligence in Financial Compliance:
In the rapidly evolving landscape of financial services, the integration of Artificial Intelligence (AI) stands at the forefront of innovation, pushing the boundaries of efficiency, accuracy, and foresight. AI’s ability to discern patterns beyond human capability, akin to distinguishing pattern predictors that heralds a transformative era for financial compliance and market predictions. This blog post delves into the impact of AI across various facets of financial services, leveraging foresight, prognostication, and projection to unveil the future interplay of AI within this dynamic sector.
AI’s super powers: above and beyond Human capabilities:
Artificial Intelligence (AI) has been a game-changer across multiple sectors, demonstrating an extraordinary capacity to identify patterns and extract insights from complex datasets beyond human capabilities. In the realm of biology, the AI system AlphaFold by DeepMind has marked a significant milestone by predicting the three-dimensional structures of proteins with remarkable precision, a breakthrough that accelerates our understanding of diseases and the development of novel therapeutics. This accomplishment, once thought to be years away, showcases the transformative potential of AI in advancing scientific knowledge.
In healthcare, AI algorithms have revolutionized the early detection of diseases by analyzing medical imagery such as X-rays, CT scans, and mammograms with higher accuracy than human experts, potentially saving lives by identifying conditions like cancer, tuberculosis, and diabetic retinopathy in their nascent stages. Similarly, the financial industry benefits from AI’s prowess in detecting fraud by scrutinizing millions of transactions in real-time to pinpoint irregularities, significantly mitigating fraud incidents. AI’s contribution extends to language processing, where models like GPT have excelled in understanding and translating languages, capturing subtle meanings, sentiments, and cultural nuances, tasks that pose challenges even for skilled human translators. This technology has facilitated communication across linguistic barriers, translating obscure languages and dialects with scant data availability.
In the environmental sector, AI models predict climate changes and model complex weather patterns, assessing the impact of human activities on the environment with unprecedented detail and accuracy. By analyzing extensive datasets from satellites, sensors, and historical records, these models offer valuable insights into future environmental conditions, guiding policy and conservation efforts. The exploration of quantum computing has also benefited from AI, which predicts the behavior of quantum systems, aiding researchers in designing more efficient quantum computers—a field where traditional computational methods and human intuition reach their limits. Moreover, in astronomy, AI algorithms process data from telescopes and space missions to unveil new celestial phenomena, including exoplanets, galaxies, and cosmic events, tasks that would be unfeasible for astronomers to perform manually due to the sheer volume of data.
AI has found applications in agriculture, where it analyzes data from satellite imagery, weather forecasts, and soil sensors to predict crop yields, detect diseases, and recommend optimal water, fertilizer, and pesticide usage. This technological intervention maximizes agricultural productivity while minimizing environmental impacts, illustrating AI’s versatile role in driving innovation and efficiency across diverse fields.
Settlement Workflow Automation: The Efficiency Frontier
The advent of AI in settlement workflows heralds a future where transactions are not only faster but virtually error-free. AI’s pattern recognition excels in identifying discrepancies in real-time, ensuring seamless reconciliation between trade orders and settlements. By automating routine checks, AI liberates human resources to tackle more complex compliance issues, enhancing operational efficiency and reducing settlement cycles significantly. The future here is not just automated but predictive, where AI anticipates and resolves bottlenecks before they impact the workflow.
As Artificial Intelligence (AI) continues to evolve and integrate more deeply into financial systems, the automation of settlement workflows is poised to redefine the efficiency frontier for brokers and financial institutions. The trajectory of AI adoption suggests a future where the settlement process not only becomes faster and more error-free but also anticipates and adapts to the dynamic nature of financial markets. Here’s how this evolution might unfold and its potential impact on brokers:
AI’s capability to analyze vast datasets in real-time will lead to the development of predictive settlement systems. These systems will not only automate current transactions but also anticipate future settlement needs based on market trends, transaction volumes, and the behavior patterns of trading partners. Predictive analytics could help in pre-allocating resources and optimizing liquidity management, ensuring that funds and securities are efficiently positioned for upcoming transactions. Reconciliation, a critical component of the settlement process, is ripe for transformation through AI. Advanced machine learning algorithms will improve the identification of discrepancies between trade orders, confirmations, and settlements. By learning from historical data, AI can predict where mismatches are likely to occur and preemptively address them, drastically reducing the time and resources spent on reconciliation.
The integration of AI into settlement workflows will significantly enhance risk management capabilities. AI systems will continuously monitor transactions for signs of operational, credit, and market risk, adjusting processes in real-time to mitigate potential issues. For brokers, this means a reduced likelihood of failed trades and the associated financial penalties, as well as enhanced protection against systemic risks. The convergence of AI with blockchain technology and smart contracts will further revolutionize settlement workflows. AI can manage and execute smart contracts on blockchain platforms, automating the settlement process with unprecedented security and transparency. This integration will reduce counterparty risks, streamline regulatory compliance, and potentially enable instant settlements, moving beyond the traditional T+1 or T+2 settlement cycles.
AI’s advancements will enable brokers to offer more personalized and strategic services to their clients. With automated settlement workflows handling the day-to-day transactions, brokers can focus on providing value-added services such as tailored investment advice, real-time market insights, and more sophisticated risk management strategies. AI can also help brokers identify new business opportunities and optimize their service offerings based on client behavior and market conditions.
As settlement workflows become more complex and automated, AI will play a crucial role in ensuring compliance with evolving regulatory standards. Automated systems will generate real-time reports, monitor compliance parameters, and even predict future regulatory changes based on legislative trends. This will not only reduce the compliance burden on brokers but also ensure that institutions can quickly adapt to new regulations. The widespread adoption of AI in settlement workflows promises to enhance the overall efficiency and stability of financial markets. Faster, more accurate, and predictive settlement processes will reduce systemic risks, improve liquidity management, and potentially lower transaction costs. However, this evolution also requires careful consideration of ethical implications, data privacy, and the potential for technological errors or biases.
As AI reshapes the landscape of financial transactions, brokers and financial institutions must stay ahead of the curve, embracing new technologies while navigating the associated challenges. The future of settlement workflows, powered by AI, holds the promise of a more efficient, secure, and responsive financial market.
Operational Efficiencies: Streamlining for the Future
Operational efficiency in financial services is paramount, and AI’s role is expanding from automation to strategic planning. Advanced analytics and machine learning models can predict peak transaction periods, optimize resource allocation, and even streamline compliance procedures by identifying patterns in historical audit data. This not only reduces operational costs but also sharpens the competitive edge of financial institutions through strategic foresight.
Stock Performance Analysis: Beyond Traditional Predictions
AI’s capability to process and analyze vast datasets enables a more nuanced approach to stock performance analysis. By examining not just financial metrics but also sentiment analysis from news articles, social media, and market trends, AI provides a holistic view of potential stock movements. This detailed analysis can enhance portfolio management, allowing for dynamic adjustments based on predictive insights rather than past performance alone.
AI has made significant strides in the domain of stock prediction, leveraging its pattern recognition capabilities and the ability to analyze vast datasets quickly and accurately. While predicting stock market movements with complete certainty is inherently challenging due to the market’s complex and speculative nature, AI has achieved notable capabilities that assist traders and investors in making more informed decisions. Here are some of the key abilities AI has developed in stock prediction:
1. Trend Analysis and Prediction
AI models, especially those using time series forecasting and machine learning algorithms, can identify patterns and trends in historical stock data. By analyzing past price movements, trading volume, and other relevant metrics, these models can forecast future trends, though with varying degrees of accuracy.
2. Sentiment Analysis
AI-powered sentiment analysis tools can sift through vast amounts of data from news articles, financial reports, social media, and other textual sources to gauge the market sentiment towards particular stocks or the overall market. Positive or negative sentiments can influence stock prices, and AI models are adept at capturing these nuances to predict potential market movements.
3. Algorithmic Trading
AI enables the development of sophisticated algorithmic trading strategies that can execute trades at optimal times based on predefined criteria. These algorithms can analyze market conditions in real time, making high-frequency trading decisions to capitalize on small price movements.
4. Risk Management
AI systems can assess and manage risk more effectively by predicting volatility and identifying potential risk factors in the investment portfolio. This allows investors to adjust their portfolios to mitigate losses and leverage opportunities.
5. Anomaly Detection
AI models are skilled at detecting anomalies in trading patterns that could indicate market manipulation, insider trading, or other irregular activities. Early detection of such anomalies can protect investments and also provide unique trading opportunities.
6. Market Impact Analysis
AI can analyze the potential impact of geopolitical events, economic announcements, and corporate news on the stock market. By understanding how similar events have historically affected stock prices, AI models can predict short-term market reactions.
7. Portfolio Optimization
Using AI, investors can optimize their portfolios by determining the best asset allocation to maximize returns while minimizing risk. AI models consider historical performance, correlations among assets, and market conditions to suggest optimal portfolio strategies.
Inflation Prediction and Reduction/Avoidance: Navigating Economic Fluctuations
The predictive prowess of AI extends to macroeconomic indicators like inflation. By aggregating global economic data, consumer trends, and monetary policies, AI models offer nuanced inflation forecasts. This foresight allows financial institutions to strategize preemptively, adjusting interest rates, investment portfolios, and loan products to mitigate inflationary impacts, embodying a proactive rather than reactive approach to economic fluctuations.
As Artificial Intelligence (AI) continues to be adopted and integrated into the financial sector, its impact on inflation prediction and management is poised to become increasingly significant. This evolution will likely manifest in several transformative ways, reshaping how financial institutions, brokers, and policymakers approach economic fluctuations and inflationary trends.
Over time, AI models will become more sophisticated through advancements in machine learning algorithms and the integration of broader and more diverse datasets. These improvements will enhance the accuracy of inflation forecasts by capturing subtle economic signals and global trends that human analysts might overlook. As AI gains the ability to analyze real-time data from a multitude of sources—including social media sentiment, geopolitical events, and even climate change impacts—it will provide a more dynamic and nuanced view of inflationary pressures.
Future AI systems will operate in near real-time, offering instantaneous analysis and predictions. This capability will transform economic monitoring from a periodic review process to a continuous one. Brokers and financial institutions will have access to immediate insights, allowing for swift adjustments to investment strategies and financial products. Real-time data processing will enable the early detection of inflationary trends, giving brokers a competitive edge in safeguarding portfolios against sudden economic shifts. As AI’s role in inflation prediction becomes more ingrained, we can expect a shift towards more automated decision-making processes. AI algorithms could be authorized to make predefined adjustments to interest rates, hedge positions, or asset allocations in response to changing inflation forecasts. This automation will reduce the latency between prediction and action, minimizing the impact of inflationary trends on investment returns and portfolio value.
The proliferation of AI in inflation forecasting will likely lead to the democratization of advanced predictive tools. Smaller brokers and financial advisors, who may not currently have the resources to develop their own AI systems, could access these technologies through third-party services or cloud-based platforms. This shift would level the playing field, allowing a broader range of actors to engage in proactive inflation management strategies. The increased reliability of AI-driven inflation predictions will not only affect brokers and financial institutions but also have significant implications for monetary policy. Central banks may begin to incorporate AI forecasts into their decision-making processes, leading to more timely and targeted adjustments to interest rates and quantitative easing measures. This could introduce a new era of monetary policy, characterized by greater precision and responsiveness to emerging economic trends.
Despite these advancements, the increasing reliance on AI for inflation prediction and management will not be without challenges. There will be concerns regarding data privacy, the potential for algorithmic bias, and the implications of automated decision-making on financial markets’ stability. Ensuring transparency, accountability, and ethical considerations in AI deployment will be crucial.
Treasury Yields and Global Bond Predictions: A New Era of Investment Strategy
AI’s application in forecasting treasury yields and global bond performance is revolutionary. By analyzing historical data, economic indicators, and geopolitical events, AI models can project future yield curves and bond market movements with remarkable accuracy. This enables investors to make informed decisions, optimizing their bond portfolios for future market conditions.
The Pattern Finding Paradigm: A New Frontier in Financial Predictions
The core of AI’s transformative potential lies in its advanced pattern finding, akin to its application in medical diagnostics. Just as AI can predict weather patterns from vast datasets, its application in financial markets promises a similar leap in predictive accuracy. By training on diverse datasets, including market trends, economic indicators, and even unrelated but influential factors like climate data, AI could forecast market movements with unprecedented precision. This goes beyond traditional analysis, offering a predictive lens through which to view potential market shifts, risks, and opportunities.
The Future: Integration, Innovation, Regulation and Ethical Considerations
As we extrapolate the future of AI in financial services, it’s clear that its integration promises a shift towards more predictive, efficient, and strategic operations. However, this future also demands a careful consideration of ethical implications, data integrity, and the need for transparent AI methodologies. Financial institutions must navigate these challenges thoughtfully, ensuring that the AI revolution in finance remains inclusive, equitable, and aligned with broader societal goals.
The increasing integration of Artificial Intelligence (AI) in financial services, particularly in forecasting and managing economic indicators like inflation, is poised to exert significant pressures on regulators and operational frameworks within the sector. The evolving landscape will likely introduce new challenges and opportunities, prompting a reevaluation of existing regulations and operational processes. Here’s an overview of the potential pressures and implications for regulators and operations:
Pressures on Regulators and Operations
As Artificial Intelligence (AI) technologies continue to advance, regulators find themselves at the forefront of ensuring that the regulatory frameworks evolve accordingly to remain relevant and effective. This evolution involves updating compliance standards to embrace the new AI-driven tools and methodologies being applied in economic forecasting and transaction management. Alongside the innovation AI brings, there are growing concerns about data privacy and security due to AI’s extensive data analysis capabilities. Regulators are tasked with the delicate balance of fostering innovation while enforcing stringent data protection regulations to safeguard sensitive financial and personal information.
Another significant challenge lies in ensuring algorithmic transparency and accountability. With AI models increasingly playing a pivotal role in decision-making processes, there’s an imperative need to establish guidelines for explainable AI. Such guidelines are crucial for ensuring that decisions made by AI systems, especially those affecting market dynamics and inflation predictions, can be understood and justified. Furthermore, the widespread adoption of AI introduces the potential for new systemic risks, including rapid market movements based on AI predictions. Regulators are required to develop mechanisms to monitor and mitigate risks associated with high-frequency, AI-driven trading and decision-making, ensuring market stability.
On the global stage, the impact of AI on financial markets calls for international cooperation to establish consistent regulatory standards. This need for cross-border regulatory harmonization arises from the global nature of financial transactions and AI applications, pressing regulators to work together to harmonize rules across jurisdictions.
Turning to the operational side, financial institutions are faced with the challenge of adapting their processes to comply with the evolving regulations regarding AI usage. This adaptation includes the implementation of new compliance checks, data handling procedures, and reporting mechanisms. Moreover, to leverage AI effectively and meet regulatory standards, firms are compelled to invest in robust technological infrastructure and continuous AI development. This necessity poses a particular strain on resources, especially for smaller firms that may struggle to keep up with the pace of technological advancements.
As AI becomes more ingrained in financial operations, the introduction of new risks becomes apparent. These include technological failures, model inaccuracies, and cyber threats, necessitating enhanced risk management strategies to address these vulnerabilities. Furthermore, the growing prominence of AI in operations signals a demand for a workforce skilled in AI technologies, data analysis, and cybersecurity. Operations are under pressure to invest in training and development to equip their teams with the necessary skills to navigate the AI-driven landscape.
Finally, there is an ethical dimension to consider. Operations must ensure that AI systems are designed and utilized ethically, avoiding biases that could lead to unfair practices or discriminatory outcomes. This ethical consideration involves implementing checks and balances to monitor AI decisions and intervene when necessary, safeguarding against potential biases and ensuring fairness and transparency in AI-driven decisions.
The AI Feedback Loop Concern
The scenario where everyone uses a highly efficient AI system for stock trading raises several interesting questions about market dynamics, the nature of trading, and the potential for systemic shifts. Here’s a breakdown of the implications and potential challenges:
The Impact on Market Dynamics
Your concern about a feedback loop where no one buys a stock predicted to go down, leaving some “holding the bag,” is valid. In theory, if all AI systems make the same predictions and guide all investors similarly, it could lead to a lack of buyers for certain stocks, causing them to drop uncontested. However, in practice:
-
- Diversity of Models: Even among high-performing AI systems, there’s likely to be diversity in predictions due to differences in algorithms, data sets, and weighting of variables. This diversity can help mitigate the risk of a universal consensus driving all market participants in the same direction.
- Adaptive and Evolving Algorithms: AI systems are not static; they continuously learn from new data. As market conditions change, so too would the AI’s predictions and strategies, potentially offsetting the risk of a static feedback loop.
The widespread adoption of advanced AI systems for stock trading and investment decision-making brings about complex implications for the financial markets. Your concerns highlight key issues related to market dynamics, the nature of trading, and the potential for systemic changes as AI becomes ubiquitous. Let’s explore these aspects:
-
- Efficiency vs. Homogenization: If every market participant uses similarly advanced AI systems, the market could theoretically become more efficient, with prices reflecting all known information almost instantly. However, this homogenization could reduce market volatility and potentially diminish trading opportunities, as AI-driven decisions converge towards similar conclusions.
- Winner and Loser Dynamics: Traditionally, markets operate on a disparity of information and judgment—some traders gain, while others lose. If all traders are guided by equally competent AI, the classic dynamic could shift. Theoretically, there might be fewer mispriced opportunities to exploit, as all participants would have access to similar levels of analysis and predictive power. This could lead to a situation where speculative gains are harder to come by, impacting the incentive structures for trading.
- Feedback Loops and Market Stability: The scenario you describe, where no buyers exist for a stock predicted to decline, could indeed create feedback loops. If all AIs predict a downturn and recommend selling or avoiding a stock, the sell-off could become self-fulfilling, potentially exacerbating market volatility. Moreover, if these AI systems act on similar signals, the market could experience sharp, synchronized movements, raising concerns about stability and flash crashes.
- Changing Patterns: As AI becomes more prevalent, the patterns on which these systems train will inevitably change. AI systems learn from historical data, but as their predictions begin to influence market behavior, the historical patterns may no longer apply, necessitating continuous adaptation of AI models. This dynamic evolution could lead to periods of unpredictability as AI systems adjust to the new patterns they themselves have helped create.
Systemic Considerations and Ethical Implications
-
- Diversity of Strategies: A healthy market ecosystem thrives on diversity—of strategies, perspectives, and time horizons. A dominance of AI could homogenize trading strategies, potentially undermining this diversity. Encouraging a range of AI approaches and maintaining human oversight could help preserve market dynamics.
- Access and Inequality: If high-performance AI systems are only available to a subset of traders or firms, this could exacerbate existing inequalities in the market. Ensuring broad access to advanced trading technologies could become a significant concern.
- Regulatory Adaptation: Regulators will need to adapt to the challenges posed by AI in trading. This might include developing new frameworks for AI transparency, ensuring systems are designed to prevent market manipulation, and monitoring systemic risks associated with synchronized AI trading actions.
While the widespread use of AI in stock trading promises increased efficiency and the potential for more informed decision-making, it also raises complex questions about market dynamics, fairness, and stability. Balancing these benefits and challenges will require careful management, ethical considerations, and perhaps new regulatory approaches. Ultimately, the impact of AI on financial markets will depend on how these systems are developed, deployed, and governed in the coming years.
The concerns raised about AI creating feedback loops in financial markets, where predictive models might lead to homogenized trading behaviors and potentially destabilize markets, touch on a broader issue known within the AI community and among systems theorists as “feedback loops” or “self-reinforcing feedback loops.” In the context of AI and machine learning, these feedback loops occur when the output of a model influences the input data used for future predictions, thereby potentially exacerbating biases, errors, or certain trends.
In financial markets, if all participants are using similar AI models for trading decisions, the models’ collective actions could indeed lead to self-reinforcing cycles. For instance, if many models predict a stock price will fall and subsequently sell that stock, the price will drop due to increased selling activity, thus validating the original prediction and potentially causing a sharper decline than might have occurred naturally.
This phenomenon is related to several concepts:
- Echo Chambers: In social media algorithms, feedback loops can create “echo chambers” or “filter bubbles,” where users are increasingly exposed to similar viewpoints, reinforcing their preexisting beliefs.
- Algorithmic Bias Amplification: In AI ethics, feedback loops are known for their role in amplifying biases. If an AI system learns from biased decisions it made in the past, it can continue to make increasingly biased decisions.
- Reflexivity in Markets: Introduced by George Soros, reflexivity refers to a feedback loop between market participants’ perceptions and the underlying market realities. While not specific to AI, the concept parallels how predictive models could influence and be influenced by market dynamics.
Addressing Feedback Loop Concerns:
- Diversity in Models and Data: Encouraging diversity in AI trading models and the data they’re trained on can help prevent homogenization of trading behaviors. If models are making decisions based on different sets of information or interpretations, the likelihood of a destabilizing feedback loop decreases.
- Adaptive Algorithms: Developing AI systems that can recognize and adapt to feedback loops by adjusting their predictive models can mitigate some of the risks. These systems need to be designed with mechanisms to detect when their predictions are influencing market behaviors unduly and adjust accordingly.
- Regulatory Oversight: Regulators can play a role by monitoring the impact of automated trading systems and imposing measures to ensure market stability. This might include stress testing AI models for feedback loop scenarios or setting limits on automated trading during periods of high volatility.
- Transparency and Collaboration: Fostering transparency among AI developers and users in the financial sector about how models are constructed and operate can aid in identifying potential feedback loop risks. Collaboration between industry players and regulators can also lead to shared standards and practices that mitigate these risks.
The AI community is increasingly aware of the complexities and unintended consequences of deploying AI in dynamic systems like financial markets. Ongoing research and dialogue are essential to understand and mitigate feedback loop concerns, ensuring that AI contributes positively to market efficiency and stability.
Changing Market Patterns
You’re correct in noting that as more AI drives decisions, the patterns themselves might change. This is a natural outcome of adaptive, learning systems operating in a dynamic environment. AI systems may initially learn from historical data, but as they begin to influence market movements, they’ll also adapt to the new patterns they help create. This could lead to an ever-evolving market landscape where AI systems continuously adjust to new data and market behaviors.
Built-in Problems and Ethical Considerations
- Market Manipulation Risks: With the widespread use of AI, there’s a potential risk for coordinated manipulation of markets. Regulatory bodies would need to stay vigilant and possibly adapt regulations to prevent such scenarios.
- Access and Inequality: If access to high-performing AI systems is uneven, it could exacerbate inequalities in the market, with well-resourced firms and individuals gaining an advantage over average investors.
- Unpredictable Systemic Risks: The collective actions of AI systems could lead to unforeseen systemic risks or market distortions. Continuous oversight and the development of countermeasures would be essential to mitigate these risks.
Conclusion: A Predictive Revolution in Finance
Artificial Intelligence in financial services is no mere fad; it’s a fundamental shift towards a future where predictive insights, operational efficiencies, and strategic foresight redefine the landscape. As financial institutions embrace AI, they unlock new dimensions of compliance, investment strategy, and market prediction, heralding an era where financial services are not just reactive but predictively aligned with the future. The journey towards this future is both exciting and challenging, promising a transformative impact on how we understand, navigate, and innovate within the financial world.
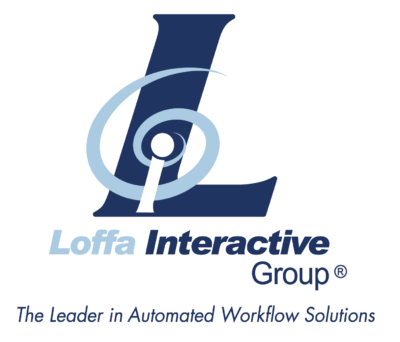